AI Model for Checking Rice Quality
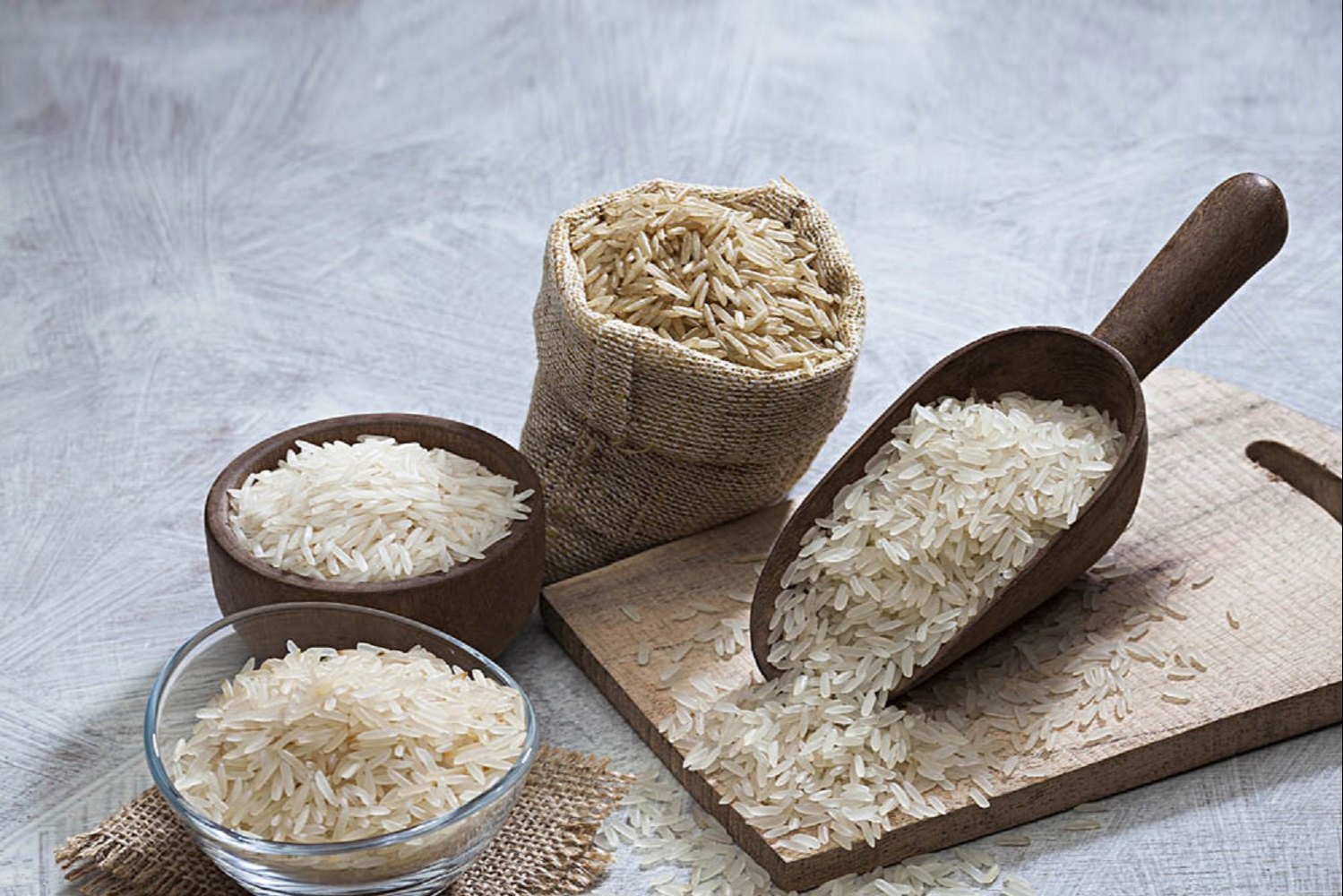
클라이언트 요구 사항
Our customer is a leading Japanese corporation that runs a chain of restaurants in eight countries with over 4000 outlets. Their goal is to become the world’s top company in the food and beverage industry. To achieve this, they need to evaluate the quality of the rice they purchase from different suppliers at various stages of the procurement process.
HBLAB의 솔루션
We used the Cascade Mask R-CNN model with general data to detect and classify rice grains into four categories: OK, error (having abnormal color), broken, and cracked.
After one month of development, we achieved the following accuracy results for each category:
프로젝트 세부정보
-
사용 기술 Instant Segmentation (Cascade mask RCNN), Synthetic data generation
-
개발팀 1 AI Engineer
-
프로젝트 기간 1 month
결과
Rice classifying AP is 97% (IOU 0,75).
Rice classification into 4 types result:
■ OK: 96,6% (AP_75)
■ Error: 86,9% (AP_75)
■ Broken: 71,1% (AP_75)
■ Cracked: 61,2% (AP_75)